Apple has published a new study demonstrating how humanoid robots can be trained more efficiently in the future. What's unique about it is that the machines no longer just learn from other robots, but directly from humans—from their perspective. This is made possible by an Apple Vision Pro, which is used for data collection. The study is called "Humanoid Policy ∼ Human Policy" and was conducted in collaboration with MIT, Carnegie Mellon, the University of Washington, and UC San Diego.
Robot training has so far been complex, expensive, and difficult to scale. Humans usually have to remotely control robots via complex teleoperation systems to teach them specific tasks. This takes time and resources. Apple is now taking a different approach: Robots are designed to learn human actions directly from videos filmed from a first-person perspective. The test person wears a headset—such as the Apple Vision Pro—and performs simple or complex actions with objects. The recordings are then used to train an AI that can instruct humanoid robots.
Ego shots instead of expensive robot data
The study investigated how human demonstrations from the first-person perspective (i.e., directly from the point of view of the person performing the action) can be used as training data. A total of over 25,000 human and 1,500 robot demonstrations were collected. This data was combined into a common format that the researchers call "PH2D." The goal was to develop a single AI policy that works based on both data sources (human and robot). The researchers emphasize that learning solely from robot data is inefficient. Acquisition is expensive, requires complex equipment, and is difficult to implement on a large scale. Egocentric human demonstrations, on the other hand, create a scalable alternative that can be used more flexibly.
How the Apple Vision Pro was used
To capture this type of training data, the researchers developed a special app for the Apple Vision Pro. It uses the headset's bottom left camera and Apple's ARKit framework to capture head and hand movements in three dimensions. This allows for first-person capture of actions—including all the motion data a robot needs to imitate the action.
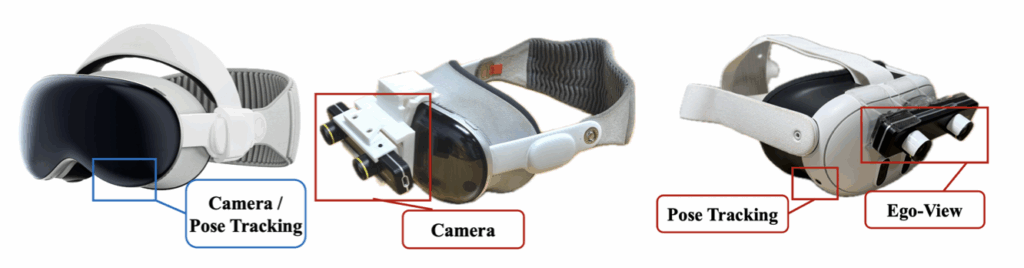
To make the method accessible to more people, a mount was also developed that allows a ZED Mini stereo camera to be attached to other headsets such as the Meta Quest 3. This allows similar 3D motion data to be captured – only at a significantly lower cost.
Faster and cheaper training
Another advantage: The training data can be collected very quickly. While traditional teleoperations often take a long time, the new method allows a complete demonstration to be recorded in just a few seconds. This not only reduces costs but also makes training much easier to scale. A side note: Since humans move much faster than robots, the recorded videos had to be artificially slowed down during the training process – by a factor of four. This allows the robots to follow human actions without the need for further adjustments.
HAT – the Human Action Transformer
The heart of the project is a model called HAT – Human Action Transformer. It was trained to process both human and robot-generated demonstrations – not separately, but together in a unified format. The advantage: HAT learns universal rules for manipulation tasks that work regardless of whether a human or a robot is demonstrating the task. Tests show that this joint training approach has helped the robot master even new, unfamiliar tasks. Compared to traditional methods, in which the robot learns only from robot examples, HAT is more flexible and requires less data.
Apple connects humans and machines in a learning model
With this study , Apple demonstrates how the training of humanoid robots can be made more efficient, cheaper, and faster. Instead of relying on complex teleoperations, the system uses simple first-person videos recorded with the Apple Vision Pro or inexpensive alternatives. The combination of human and robot-generated data in a common model is the key advancement. Anyone interested in robotics or artificial intelligence will gain an exciting insight into the future of machine learning with this work—with Apple as one of the drivers of this development. (Image: Shutterstock / Golden Dayz)
- Apple completely restructures secret robotics team
- Foxconn: AI and robotics take over the assembly lines
- OpenAI acquires Jony Ives startup for new AI devices
- Google I/O: These are the most exciting announcements of 2025
- How stolen iPhones are smuggled to China worldwide